Capturing knowledge of manufacturing process capability from design data
This research introduces a data-driven framework for automating the acquisition of manufacturing process capabilities essential for on-demand cyber manufacturing. The framework follows two paths in parallel: it uses deep learning and Convolutional Neural Nets to identify features in parts (e.g., holes, gear teeth); it employs a sequence mining algorithm to identify common process sequences that realize parts.
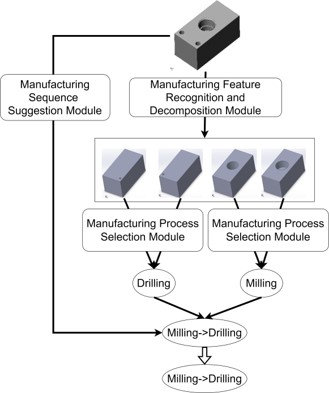
Part data is a vector described with shape signatures (a compact abstraction of a shape for easier comparison, e.g., the distribution of pairwise distances of mesh vertices, aka. D2 distribution), material properties, and part quality metrics.
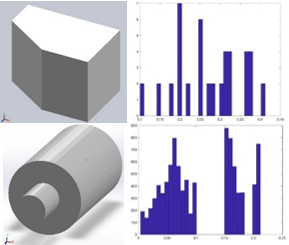
The framework demonstrates high accuracy and confidence, indicating its predictive strength for Computer Aided Process Planning (CAPP). Additionally, we have explored various shape descriptors for similarity search in previously manufactured parts, comparing non-machine learning methods like D2 distribution and spherical harmonics with machine learning methods using different representations of 3D part models. We have found that different shape signatures perform better with certain geometric representations, e.g., spherical harmonics perform best in general, while deep learning on point cloud excels for rotational invariance (suggestions do not depend on how the part sits in a coordinate system).
Furthermore, we have found that instead of determining how a new part should be manufactured as a classification, we can search for similar parts that exist in a database of previously manufactured parts, with the rationale is that a candidate manufacturer should nominally have produced parts with similar function and manufacturing process requirements. Similar parts can be retrieved by searching for the closest latent vector in the database, where the vector is formed after training a Variational Auto-Encoder where the input and output to a neural network are the same. The proposed method outperforms shape-only retrieval models and supervised learning models trained with either process or functional labels.
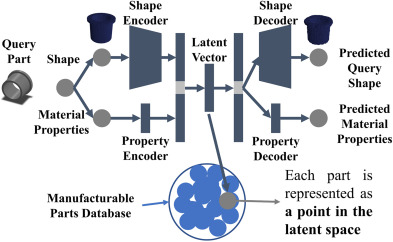
Creating an ontology of design for additive manufacturing
Additive manufacturing gives designers new freedoms to create complex geometries and combine parts into one. However, it has its own limitations, and more importantly, there are less mature guidelines for designers to adopt. To formalize knowledge of design for additive manufacturing processes, we have developed an ontology using formal RDF and OWL representations in the Protégé tool. The knowledge is represented in terms of a set of subjects (abstract entities), object properties (relations or links among the entities). The subjects have a hierarchical structure where subclasses have a standard RDF:isA relationship with the superclass. This formal modeling approach also includes defining data properties (attributes of entities), cardinality rules, types of object properties (e.g., symmetric or directional relations), properties of subject sets (e.g., an entity being disjoint with other entities under a superclass), and cardinality rules. We demonstrate how information about building three types of design features were instilled in different modeling attempts. The features are revolute joint, thread assembly (screw connection), and slider-crank. How multiple instances (build events) are stored and retrieved in the knowledge base is discussed in light of different modeling decisions that were made in different versions of the ontology. A set of competency questions are described which help evaluate the sufficiency of the ontologies. Examples are given about how queries are formalized in SPARQL (the formal querying language for semantic web) and what can be retrieved. The formalized ontology not only provides a way to capture and describe meta-knowledge about design for additive manufacturing, but also creates a basis for documenting the existing ad-hoc information in the literature on guidelines for how to utilize the different additive processes and what their limitations are. Knowledge documentation is the main objective of the current ontology. However, representation of design rules in a structured modular hierarchy, and reasoning about these rules in addition to discovering new rules (e.g., generalizations with induction) are future directions and considered when modeling current ontologies.
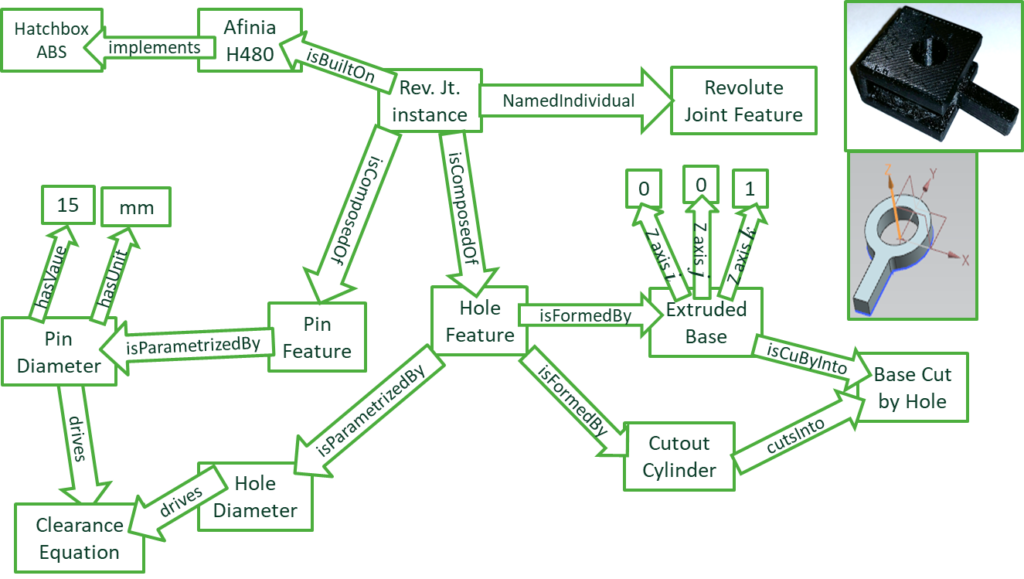
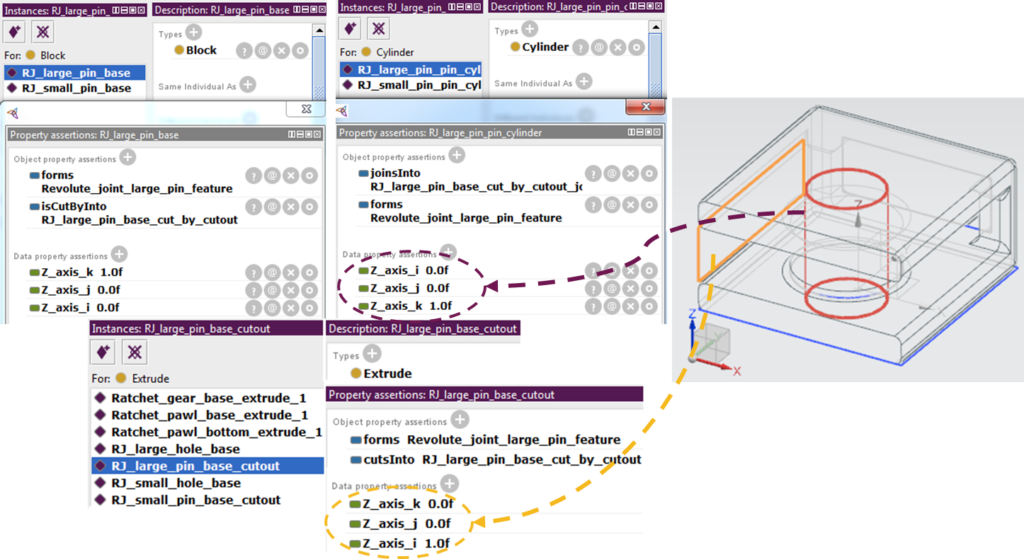
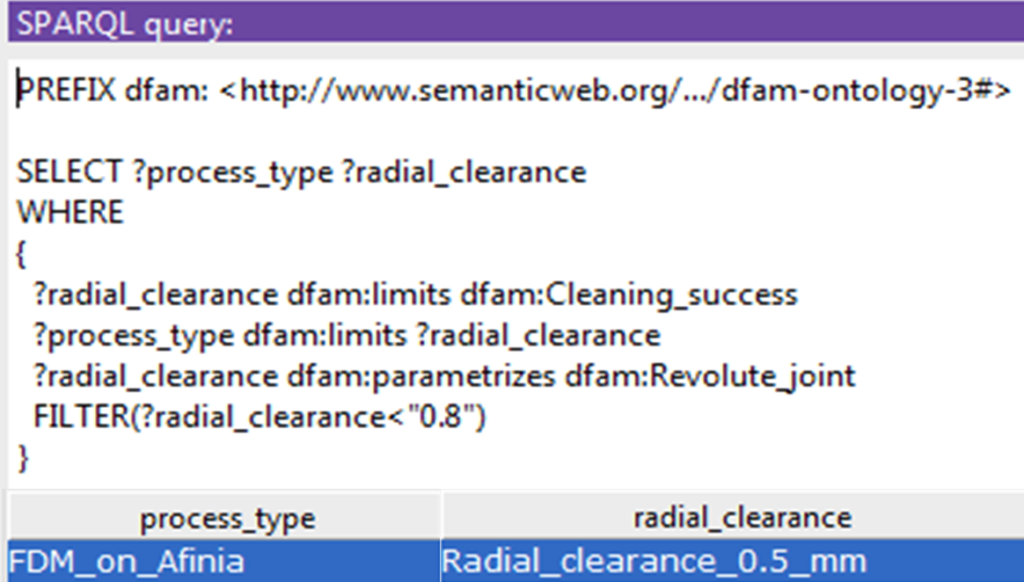